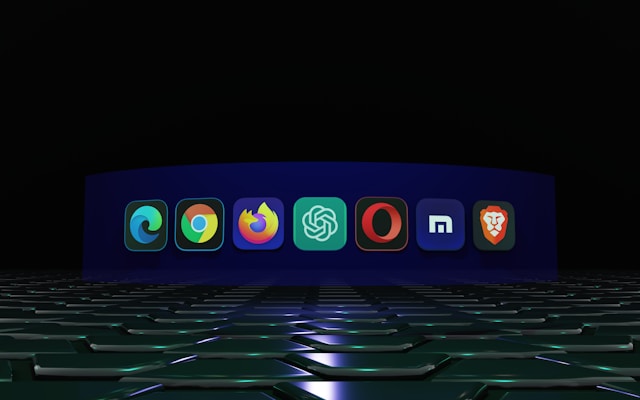
What Is An AI Developer?
Discover what an AI developer does, the skills required, how they contribute to AI-driven projects and learn why AI developers are essential in today’s tech landscape!
Read More
Artificial intelligence is transforming industries, but building AI solutions often comes with a pretty high price tag. We get it - it’s tempting to deem it a trend and pass out on its benefits since the costs can easily skyrocket with this kind of new technology. Between data acquisition, model development, and deployment, the price of AI development can quickly spiral out of control. For many businesses, these financial blockers make scaling or even starting AI initiatives seem out of reach.
The good news? At DevelopersLATAM, we’re certain that with the right strategies, you can cut costs without sacrificing quality. From leveraging open-source tools to outsourcing development to cost-effective regions, there are proven ways to reduce expenses while staying competitive. This article will walk you through eight practical strategies to make AI development processes more affordable. Whether starting from scratch or optimizing an existing project, these tips will help you maximize ROI and minimize spending. Let’s dive right in, shall we?
AI development can be expensive, and understanding why costs escalate is crucial for managing your budget. Several factors contribute to these rising expenses, often catching businesses off guard. But you don’t want this to be the case for your organization, which is why you need to conduct a thorough assessment of every potential detail that might lead to your company incurring higher-than-necessary AI development costs. Here are a few:
You’ve heard the news: data is the backbone of AI… but acquiring and preparing it isn’t cheap. Collecting high-quality data often involves licensing fees or purchasing datasets, which can cost anywhere from $1,000 to $100,000, depending on the industry. On top of that, data cleaning and labeling - essential for training AI models - require significant resources. A report by Cognilytica notes that data labeling costs can range from $0.05 to $0.50 per label, which adds up quickly when dealing with millions of data points.
Developing custom AI models often requires specialized expertise and tools, both of which come with steep costs. According to Glassdoor, hiring skilled AI developers can cost an average of $100,000 to $150,000 annually per developer in the US Additionally, building models from scratch involves trial and error, which extends timelines and inflates budgets. Businesses frequently underestimate how long it takes to test and refine models, leading to unplanned expenses.
Running AI models demands powerful computing infrastructure. Training a large AI model can require servers equipped with high-performance GPUs, costing $5,000 to $10,000 per server annually. Cloud providers offer scalable solutions, but their fees add up over time. For instance, training OpenAI's GPT-3 reportedly cost millions of dollars in cloud computing resources. While your project might not reach this scale, infrastructure expenses are still a significant budget item.
Once deployed, AI systems don’t maintain themselves. They require ongoing monitoring to ensure performance doesn’t degrade. Model retraining is often necessary as data changes or new use cases arise. According to Deloitte, maintenance can account for 40% to 60% of an AI project’s total lifecycle costs. Without proper planning, these expenses can cripple your budget.
AI development costs in 2024 are shaped by growing demand, technological advancements, and market dynamics. Understanding current cost drivers can help you plan your budget and avoid unexpected expenses. Here’s why AI costs have increased this year!
The talent shortage in AI development continues to drive up costs. The average salary for a machine learning engineer in the U.S. ranges from $120,000 to $160,000 annually, according to LinkedIn's 2024 workforce report. In regions like Europe, Latin America, and Asia, salaries are lower but still higher than other IT roles. Contracting freelance AI developers can cost $75 to $150 per hour, depending on experience and specialization. As demand for these roles grows, competition for skilled professionals will keep costs high.
AI projects increasingly rely on powerful computing resources. Training a state-of-the-art AI model, such as a large language model, can cost $500,000 to $1.5 million in cloud services alone, according to industry estimates. Even for smaller projects, GPU-based cloud instances cost $3 to $10 per hour on platforms like Azure. These expenses multiply quickly during model training and deployment phases.
Data remains one of the most significant costs in AI development. Acquiring proprietary datasets can range from a few thousand dollars to millions, depending on the industry. For instance, healthcare data or financial transaction datasets often carry premium price tags due to regulatory requirements and confidentiality. Preparing this data for AI—cleaning, labeling, and organizing it—can consume up to 80% of the total project budget, according to Forbes.
As regulations surrounding AI evolve, compliance is becoming a cost driver. In 2024, businesses developing AI systems in regions like the EU must adhere to frameworks like the EU AI Act. This includes auditing data sources, ensuring model transparency, and implementing safeguards against bias. Meeting these requirements often requires additional legal consultation, technical assessments, and system adjustments, adding to overall costs.
While pre-trained AI models and APIs offer cost-effective solutions, customization is often necessary to meet specific business needs. Custom AI development typically costs between $50,000 and $250,000 per project, depending on complexity, according to Deloitte's 2024 AI trends report. Companies seeking competitive advantages through tailored AI solutions must prepare for these higher upfront costs.
The size and complexity of your AI project directly affect its cost. A simple chatbot will cost far less than a custom-built predictive analytics tool. According to a Deloitte report, AI projects with highly customized requirements can cost 5 to 10 times more than off-the-shelf solutions. Defining clear goals and deliverables can help you control scope creep, which often leads to inflated budgets.
Data is the foundation of any AI system, but sourcing and preparing it can vary in cost. Proprietary data acquisition, data cleaning, and labeling all contribute to overall expenses. For example, building a dataset for image recognition might involve labeling thousands of images, which can cost $0.10 to $0.50 per label depending on complexity. Assess whether your project can leverage existing datasets or requires significant investment in data preparation.
The tools and platforms you choose play a critical role in cost management. Open-source frameworks like TensorFlow and PyTorch can reduce licensing fees, but implementing them may require in-house expertise. On the other hand, turnkey solutions from cloud providers like AWS offer convenience but come with ongoing subscription costs. Balancing affordability with functionality is key.
AI development often requires specialized skills that don’t come cheap. Hiring an experienced AI developer in the US costs an average of $120,000 to $150,000 annually, according to Glassdoor. Outsourcing to nearshore or offshore locations can reduce costs significantly, but it’s important to factor in communication challenges and potential time zone differences.
Running AI models demands significant computational power. Cloud computing services charge based on usage, with GPU instances costing $3 to $10 per hour depending on the provider. For larger projects, investing in on-premises infrastructure may offer long-term savings, but the upfront cost can be substantial. Evaluate your project’s computational needs to choose the most cost-effective solution.
AI development costs don’t end at deployment. Models need regular updates, retraining, and monitoring to remain effective. Maintenance can account for up to 60% of the total cost of ownership, according to Accenture. Planning for these ongoing expenses from the outset helps you avoid budget overruns later.
Begin with a minimum viable product (MVP) to test your AI system's feasibility before committing to full-scale development. This approach minimizes upfront costs and allows you to identify potential issues early. A McKinsey study found that companies using MVPs in AI projects reduced their initial investment by up to 40%, reallocating resources to refine and scale successful models.
Leverage open-source frameworks to save on licensing fees. These tools are widely supported by a global developer community, ensuring regular updates and compatibility with cutting-edge technologies. According to O’Reilly’s 2024 AI Trends Report, using open-source platforms can cut AI development costs by 25% compared to proprietary software.
Partnering with nearshore or offshore teams can significantly reduce labor expenses while maintaining quality. For example, AI developers in LATAM or Eastern Europe often charge 40–60% less than their U.S. counterparts while offering similar expertise. Outsourcing also allows you to scale your team flexibly, paying only for the resources you need.
Instead of acquiring expensive proprietary datasets, explore alternative sources like publicly available data or synthetic data generation. Synthetic data can reduce costs by up to 80%, according to Gartner, while still delivering accurate training results. Streamlining data cleaning and labeling processes with automation tools can also cut expenses.
Pre-trained models such as OpenAI’s GPT series or Google’s BERT can save significant development time and money. Customizing these models for your use case is often more cost-effective than building one from scratch. In fact, adopting pre-trained models can save up to 90% of development time and costs, according to a report by McKinsey.
Cloud platforms offer scalable solutions, but overprovisioning resources can lead to unnecessary expenses. Monitor your cloud usage carefully, and take advantage of features like auto-scaling to avoid paying for idle resources.
Manual testing is both time-consuming and costly. Automating testing and debugging with tools like Selenium or Test.ai can accelerate development and reduce errors. The initial investment in automation tools often pays off quickly, with up to a 30% reduction in overall testing costs, according to a study by Capgemini.
Training existing team members in AI tools and methodologies can be more cost-effective than hiring new talent. Platforms like Coursera and Udemy offer affordable courses on AI and machine learning. Upskilling your team not only saves hiring costs but also fosters a deeper understanding of your project’s unique requirements.
Reducing AI development costs doesn’t just mean cutting corners. On the contrary, it’s all about working smarter to achieve the best results with your resources. By prioritizing strategic actions like leveraging pre-trained models, optimizing cloud usage, and outsourcing wisely, businesses can lower expenses while still delivering impactful AI solutions.
If you’re looking for a reliable partner to streamline your AI development processes, or your company is in need of talented developers, at DevelopersLATAM we are more than ready to jump in. We can offer you dedicated Nearshore Staff Augmentation and Custom Software Development cost-effective solutions tailored to your needs with our many years of experience. With our help, you can access top AI development talent across Latin America and reduce costs without compromising quality. Ready to get on top of the AI market? Contact us right now to schedule a discovery call and begin the journey toward technological success!